Sales
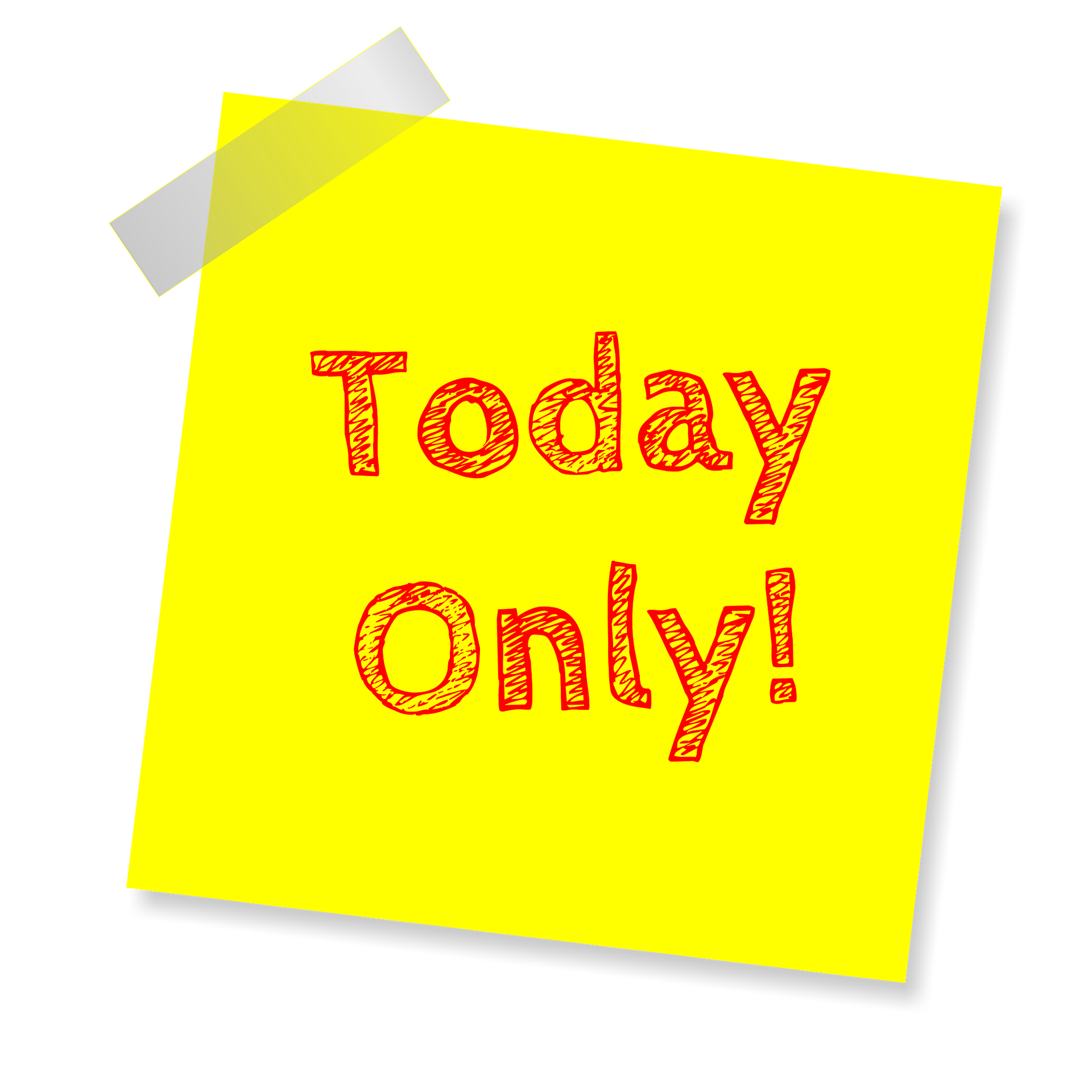
Market basket analysis -AFFINITY
Product recommendation:
“Customers who bought/viewed this item also bought/viewed…”
- Understand the purchase behaviour of buyers, their needs, to better prepare promotional programs (what products are most frequently sold together).
- One can divide customers into groups or divide products into groups.
- By selecting a profile of customers, say teenager girls, one can scrutinize what (combination of) products they buy or visit in a website. By selecting a specific product, say vegetarian (no) beef burger, one can get a profile of the buyers (demographic data).
Demand forecasting and price optimization
Both are challenging projects and many factors are to be considered to predict units to be sold at different prices. The most sensible thing to do is to address one factor at a time using different tools. Below are examples of data preparation prior to any “smart” statistical approach.
Different event lengths overlapping each other (maybe be due to Flash sales or just additional discounts to boost sales sooner than expected) will change the planned number of days at a price (no overlap was anticipated): adjusting a planned duration into a real duration is not immediate when handling thousands of SKU (Stock keeping units). Without this adjustment, the investigation will not be reliable.
- If items (SKU) are defined by “equal price”, what does “stock-out” mean? imagine a collection of 20 lipsticks, if the fashion colour is sold-out, the whole collection is sold-out? New price will mean price for non-fashion colours: no “demand curves” to compute since here it is assumed the item is the same. Clothing sizes have a similar problem because the price tends to be the same across sizes, but no concern for parfums because different sizes have different prices.
- “Zero units sold” at a price are fundamental but they are not in Sales datasets unless they are included on purpose.